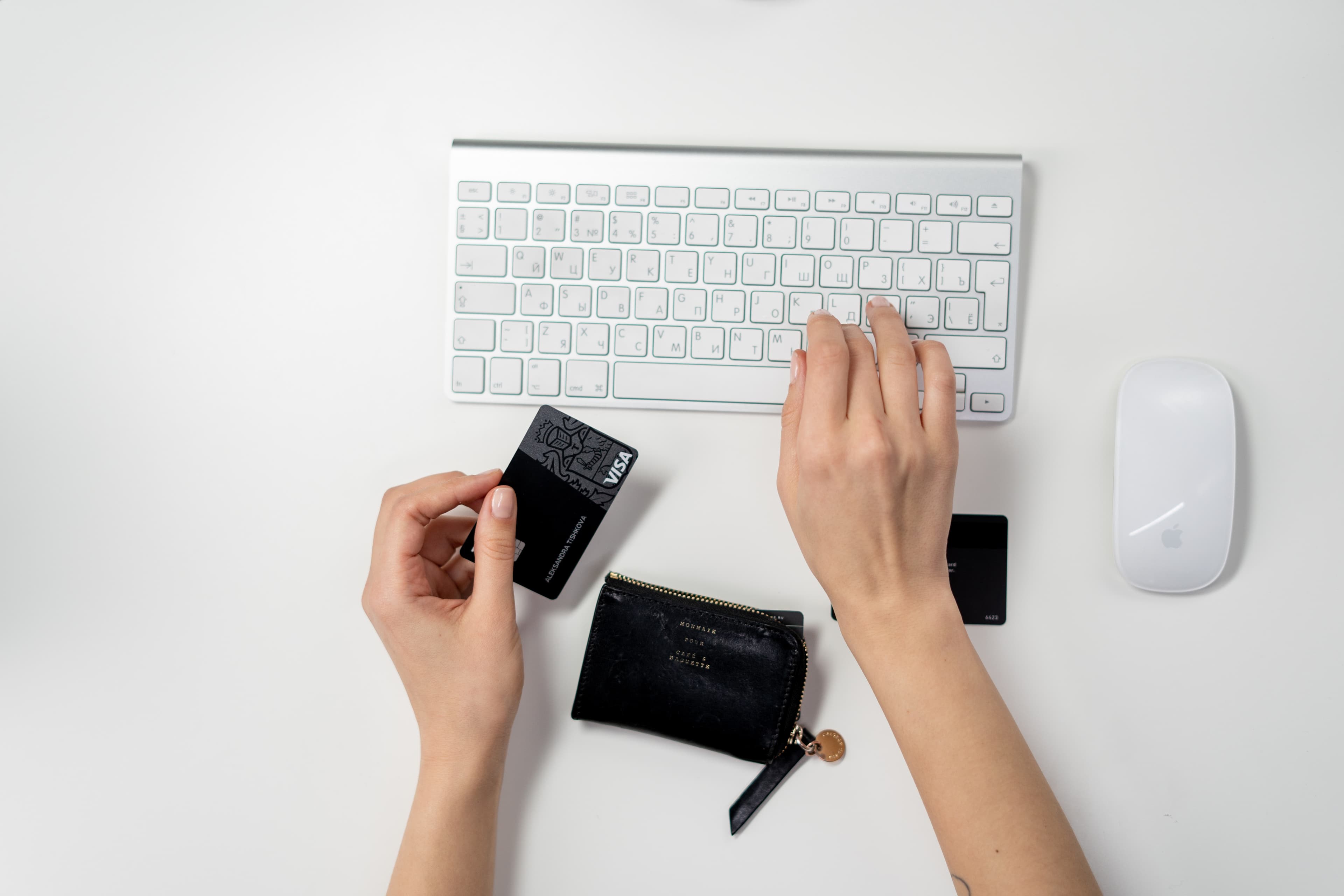
23.5.2023
Machine Learning in Sales Prediction: The Next Wave of Forecasting
Reine Amabel Jaruda, Freelance Writer for OctoShrew
Introduction
Sales forecasting is an important part of any business. It helps you understand what products to make and how much stock to keep on hand. Traditionally, this has been done through manual processes and formulas. However, machine learning is changing the game. In this post, we'll explore how machine learning can be used in sales forecasting and the benefits this brings.
How is ML used for sales prediction?
Machine learning has been used in a variety of ways to predict outcomes. One common use is to predict whether or not a customer will make a purchase. This is done by analyzing data about past customers and their behavior. The data can include anything from how often a customer makes a purchase to what type of products they are likely to buy. Marketing campaigns and the development of products can even also be generated through the use of ML and AI.
By analyzing data about past customers and their spending habits, machine learning can help businesses develop a better understanding of how likely a customer is to spend a certain amount of money. This information can be used to create targeted marketing campaigns and to develop products that are more likely to appeal to certain customers. In fact, machine learning has been shown to be more accurate that human forecasts. Traditional forecasting methods often used to rely on human intuition and experience to make predictions, however, ML can spot trends that individuals would not normally be able to see.
ML allows us to can make better decisions about where to allocate their resources. It can also help us to identify opportunities and threats in the market.
Best Algorithms for Sales Prediction
When it comes to sales prediction, there is no one-size-fits-all algorithm. Choosing the best algorithm for your data and business needs is essential for getting accurate predictions.
Some of the most popular algorithms for sales prediction include the following:
Regression analysis:
Through regression analysis, one can predict the effects of an independent variable based on a dependent one. In sales, this is useful for predicting future sales based on historical data.
Decision Trees:
The algorithm creates a tree-like model of decisions and their possible consequendces based on certain variables. Decision trees are most useful for determining customer behavior and predict which products would be bestsellers.
Random forests: Random forests are a type of machine learning algorithm that are used to predict outcomes based on historical data. Like decision trees, they can be used to predict the likelihood of future sales.
Neural Networks
This is a machine learning algorithm that is inspired by the structure and function of the human brain. Neural networks are able to learn and make predictions based on complex patterns in data hence they are good for sales prediction.
Applications of ML in sales forecasting
Machine learning truly is making huge advancements in sales prediction and forecasting. In fact, businesses themselves are beginning to realize the benefits of using this technology to improve their forecasting accuracy.
Some of the most common applications of machine learning in sales prediction are demand forecasting and customer segmentation. Demand forecasting uses historical data to predict future demand for a product or service. This information can be used to make decisions about production planning, inventory management, and pricing.
Customer segmentation is the process of dividing customers into groups based on their characteristics, needs, and behavior. This information can be used to create targeted marketing campaigns and to improve the customer experience.
Pros and cons of ML in sales forecasting
The potential of machine learning has the revolutionize sales forecasting. Its ability to automatically learn and improve from data makes it a powerful tool for predicting future outcomes. However, with all most things, ML has it own set of drawbacks.
Here are some of the pros and cons of using machine learning for sales forecasting:
Pros of machine learning in sales forecasting:
-
Machine learning can automatically learn and improve from data which makes it a powerful tool for predicting future outcomes.
-
Machine learning can identify patterns and relationships in data that humans may not be able to see.
-
Machine learning can help sales organizations optimize their sales process and improve their forecasting accuracy.
-
Machine learning can help sales organizations identify and respond to changes in the market more quickly.
Cons of machine learning in sales forecasting:
-
Machine learning can be expensive and time-consuming to set up and maintain.
-
Machine learning algorithms may not be able to identify all the patterns and relationships in data.
-
Machine learning can be inaccurate if the data is not representative of the real world.
-
Machine learning can be biased if the data is not representative of the population.
Conclusion
The potential for machine learning in sales prediction is huge. It can be used to predict a wide variety of outcomes, including customer behavior, spending habits, and future trends, among others. Because of this, it can help salespeople allocate their time and resources more effectively and efficiently.
Moving forward, the next wave of forecasting will be to use machine learning in making predictions about future sales.
Related blogs
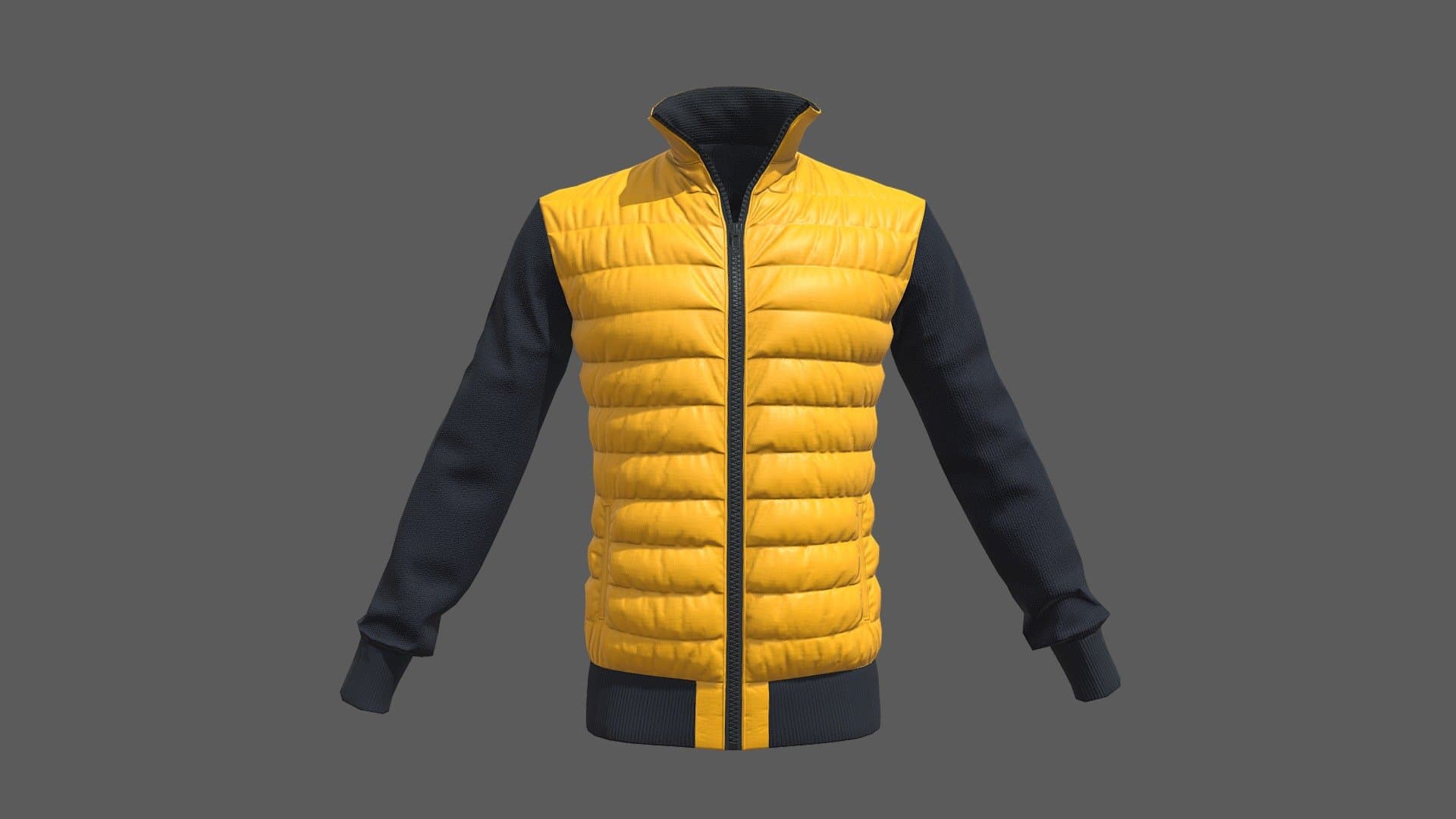
23.5.2023
3D Clothing Reconstruction for Online Marketplaces
Mayank Laddha, Machine Learning Engineer @ OctoShrew
case-study, shopping, deep-learning, computer-vision, machine-learning
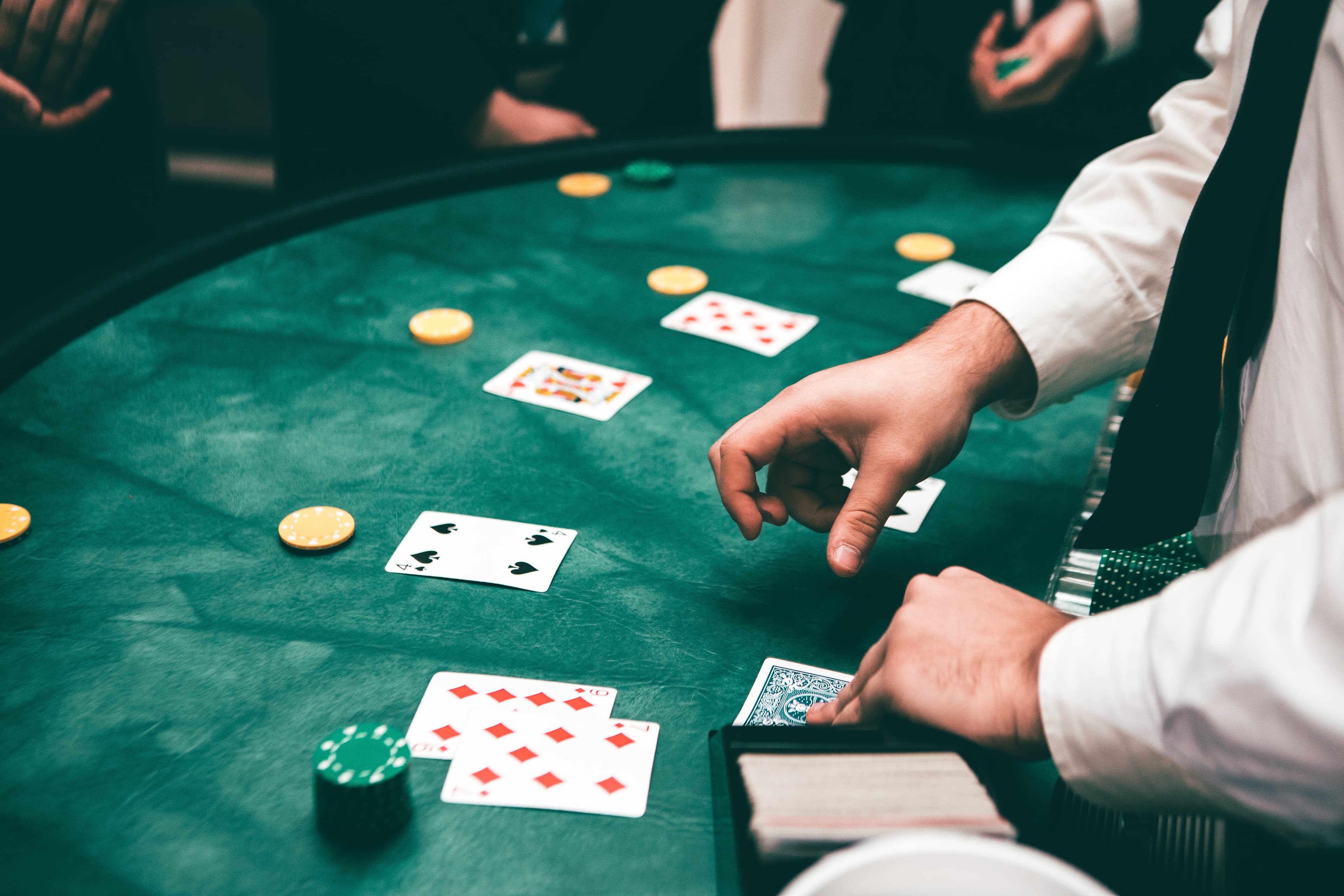
23.5.2023
Blackjack Surveillance for detecting Card Counters
Szymon Fonau, CTO @ OctoShrew
case-study, data-science, computer-vision
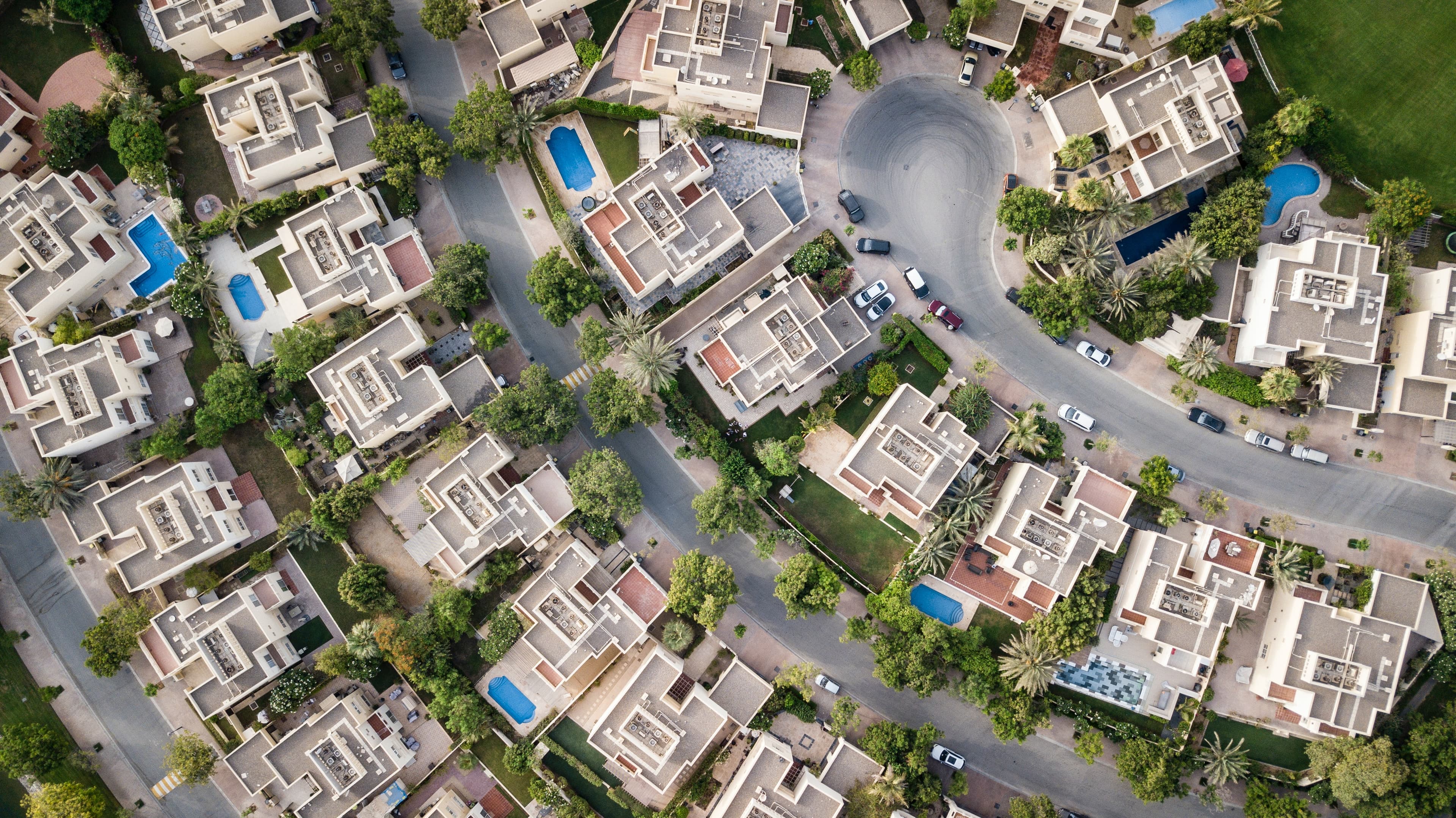
23.5.2023
Boosting Conversions with Automated House Value Estimation
Szymon Fonau, CTO @ OctoShrew
case-study, data-science, marketing, machine-learning